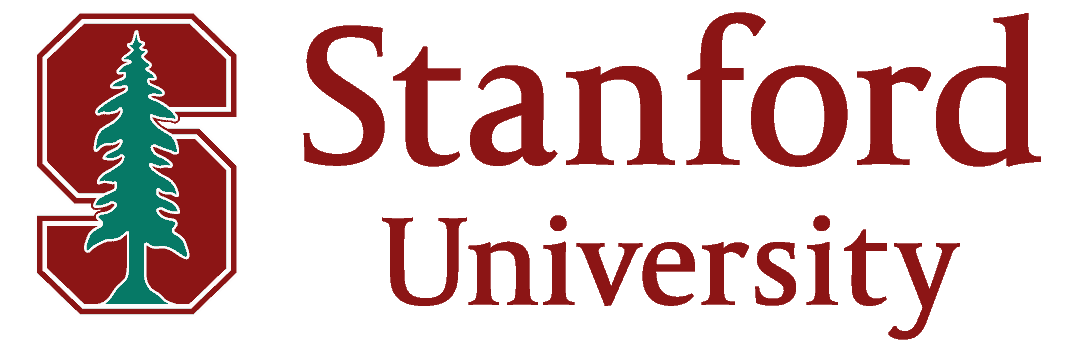
ManiWAV:
Learning Robot Manipulation from In-the-Wild Audio-Visual Data
Zeyi Liu1    Cheng Chi1,2
   Eric Cousineau3    Naveen Kuppuswamy3   
Benjamin Burchfiel3    Shuran Song1,2
1Stanford University      2Columbia University      3Toyota Research Institute
Audio signals provide rich information for the robot interaction and object properties through contact. These information can surprisingly ease the learning of contact-rich robot manipulation skills, especially when the visual information alone is ambiguous or incomplete. However, the usage of audio data in robot manipulation has been constrained to teleoperated demonstrations collected by either attaching a microphone to the robot or object, which significantly limits its usage in robot learning pipelines. In this work, we introduce ManiWAV: an 'ear-in-hand' data collection device to collect in-the-wild human demonstrations with synchronous audio and visual feedback, and a corresponding policy interface to learn robot manipulation policy directly from the demonstrations. We demonstrate the capabilities of our system through four contact-rich manipulation tasks that require either passively sensing the contact events and modes, or actively sensing the object surface materials and states. In addition, we show that our system can generalize to unseen in-the-wild environments, by learning from diverse in-the-wild human demonstrations.
Technical Summary Video (4 min)
Capability Experiments
(a) Wiping Whiteboard 🪧
The robot is tasked to wipe a shape (e.g. heart, square) drawn on a whiteboard. The robot can start in any initial configuration above the whiteboard and grasp an eraser in parallel to the board. The main challenge of the task is that the robot needs to exert a reasonable amount of contact force on the whiteboard while moving the eraser along the shape.ManiWAV (unmute to hear the contact mic recording):
Baselines:
Key Findings:
- Incorporating contact audio as additional source of information improves robustness and generalizability of the policy.
- Noise augmentation is an effective strategy to bridge audio domain gap between human collected data and robot deployment data, and increase the system's robustness to out-of-distribution sounds.
- Transformer encoder is better for fusing the vision and audio features comparing to using MLP layers.
(b) Flipping Bagel 🥯
The robot is tasked to flip a bagel in a pan from facing down to facing upward using a spatula. To perform this task successfully, the robot needs to sense and switch between different contact modes -- precisely insert the spatula between the bagel and the pan, maintain the contact while sliding, and start to tilt up the spatula when the bagel is in contact with the edge of the pan.ManiWAV (unmute to hear the contact mic recording):
Baselines:
In-the-Wild Generalization:
Key Findings:
- Action diffusion yields better behavior comparing to MLP policy model.
- Training a transformer audio encoder from scratch yields better performance comparing to using a CNN-based encoder.
- In-the-wild data enables generalization to unseen in-the-wild environments.
(c) Pouring 🎲
The robot is tasked to pick up the white cup and pour dice out to the pink cup if the white cup is not empty. When finish pouring, the robot needs to place the empty cup down to a designated location. The challenge of the task is that the robot cannot observe whether there are dice in the cup or not given the camera view point both before and after the pouring action, therefore it needs to leverage feedback from vibrations of objects inside the cup. Watch the below video for details of the task and ablations.Key Findings:
- Audio can provide critical state information beyond visual observations.
- Policy performance is sensitive to audio history length, either too short or too long will hurt performance.
(d) Taping Wires with Velcro Tape âž°
The robot is tasked to choose the 'hook' tape from several tapes (either 'hook' or 'loop') and strap wires by attaching the 'hook' tape to a 'loop' tape underneath the wires. The challenge of the task is that the difference between 'loop' and 'hook' tape are not observable with vision, but the subtle difference in surface material can generate different sounds when 'sliding' the gripper finger against the tape. Watch the below video for details of the task and ablations.Key Findings:
- Contact microphone is sufficiently sensitive to different surface materials.
- Training-time noise augmentation is more effective than test-time noise reduction.
More Results
Attention Map Visualization
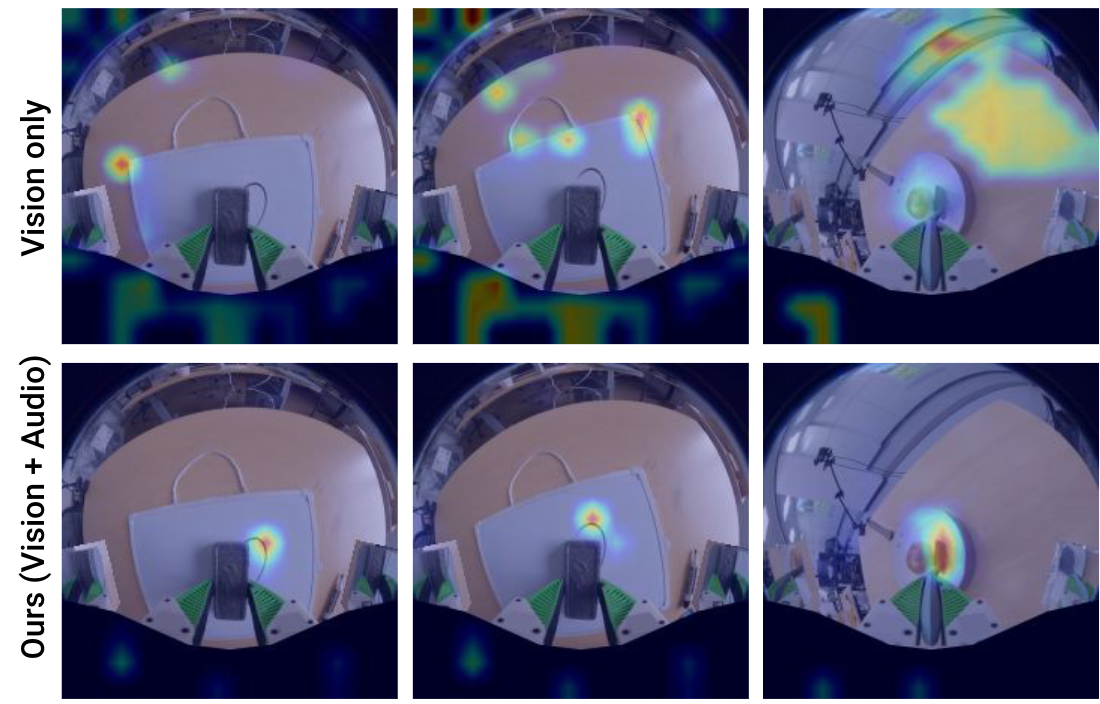
Interestingly, we find that a policy co-trained with audio attends more on the task-relevant regions (shape of drawing or free space inside the pan). In contrast, the vision only policy often overfits to background structures as an shortcut to estimate contact (e.g., the edge of the whiteboard, table, and room structures).